How do you best learn data science and then get a job? How long does it take, and how much does it cost? What is data science? There are so many questions you guys have been asking me, so in this article, I thought I would try to answer them and share the three best ways to achieve your goal of getting a data science job and Since there’s no simple answer, It’s really important to have a look at all three methods so that I can help you pick the best choice for yourself.
Ok, let’s get started. All the way back in 2012, Harvard Business Review said that data science was the sexiest job of the 21st century and recently followed up with an updated version of their article. So before we look at how to learn data science, we need to know what data science really is. I mean, ML engineers often spend most of their time handling and understanding data, right? So how is a data scientist different from an ML engineer? Well, there are three reasons for this confusing overlap between the role of a data scientist and the role of an ML engineer. First, if we look at Wikipedia’s definition of data science, we see that it says data science is an interdisciplinary academic field that uses statistics, scientific computing, scientific methods, processes, algorithms, and systems to extract or extrapolate knowledge and insights from potentially noisy, structured, or unstructured data. Well, all of that is more or less just a more confusing way of saying that data scientists use data to find some patterns so that they can make some predictions. And since machine learning models learn from data and can extract and extrapolate knowledge, machine learning is part of data science. Second, and this is very important, traditionally, companies have data science teams to generate business insights from data, which can be done using machine learning. Finally, the mentioned extrapolation of knowledge, as mentioned, pretty much just means predicting some behavior. For example, forecasting customer demand. And this, of course, can be done using machine models. In the end, the goal of data science is to generate business insights, whereas the goal of ML engineers is to turn data into products. This means that data scientists deal much more with understanding and interpreting the data and tend to be better statisticians, while ML engineers tend to be better engineers. Also, ML engineers definitely need to know machine learning algorithms.
But many data scientists can do their job without ever touching ML. It’s all about the data and business insights you get where you potentially want to apply machine learning. And with the increasing number of pre-built and pre-trained models that can work off the shelf, it is likely that many data scientists will have to do even less actual machine learning engineering and require less complex ML knowledge. So now that you have a better idea of what data science is, let’s have a look at the three ways you can learn data science and figure out which one is best for you. So I’ve spent the past 5 years studying computer science at college, and I definitely don’t regret it. But there certainly are pros and cons to going down this route that might be deal-breakers for you. Back when I started, I had no idea what to learn or how to get started. And attending a college, where people have laid out a specific 3-5 year curriculum for a bachelor’s and master’s program, was the easiest way to get started. I didn’t have to think of what might be relevant and what was not, because, just like in school, people told me what to learn. And in general, most of what I learned was indeed useful, especially for exploring the different directions you can go with computer science. But that said, I would argue that depending on what you want to achieve, it might be completely overkill. If you already know that you want to work in data science, there will be a lot of additional courses that are not as important to study in that much depth, at least. And spending 3-5 years learning the specific skills you need is a very long time, requires a lot of commitment since it is a full-time degree, and is arguably very expensive. Now, I agree that a degree is still the most broadly accepted way to prepare for a job and get the skills you need for a job, but that is changing every year. In the end, companies care about whether you can do the job or not. Even Google does not explicitly require a master’s or bachelor’s degree for a data science job, but they want equivalent experience. Now, the biggest advantage I see with colleges is the people and connections you make. You can much more easily find people who are way ahead of you that you can learn from. People with internships and jobs at amazing companies can even perhaps refer you. PhD students or even professors that can perhaps mentor you and have connections to other great companies, both of which are insanely valuable. And it’s simply more fun to study with friends.
I personally have met amazing people at college and have amazing mentors slash advisors. So if you can afford to go to college, be it time- or money-wise, and you think what I just said sounds amazing, I can definitely recommend going to college. But what if you are not 18 years old and don’t have the time to commit to studying full-time for three to five years? Perhaps you already have a bachelor’s degree and want to pivot, or you simply don’t like the way colleges teach. There are so many reasons why college is overkill, depending on your situation. I mean, again, in the end, it comes down to the specific skills required for a data science job and being able to prove that you have those skills. Simply put, colleges are no job guarantee; expect perks at MIT, Stanford, and so on, but honestly, even what you learn at college is not exclusive to college; pretty much all you learn is available online, arguably taught even better. That means college might not be the right choice in your case. So let’s have a look at the next option to learn data science. Okay, so what if you want to jump to the complete opposite of what I’ve just described? What if you can’t commit to the 40-hour-per-week job of being a student and don’t have the money to pay expensive tuition fees and perhaps even relocate? Well, there, of course, is the option of being self-taught, which again has pros and cons, and you have to consider all of them. First of all, as mentioned before, pretty much everything you would learn in college Exists somewhere on the internet for free or at a much cheaper price. You might end up buying an ML course. But that might only be a couple of tens or hundreds of dollars. Another great thing is that you have the freedom to schedule your learning however suits you. You can’t treat it like a full-time job studying every day, but you can also adjust it a bit to your life by studying in the evenings or on the weekends. But that can also be an issue, because being self-taught is very unstructured. It is very difficult to construct your own detailed curriculum that will teach you everything you need to know and help you build a portfolio. A great portfolio is even more important for you as a self-taught person because you don’t have the credibility of having graduated from college.
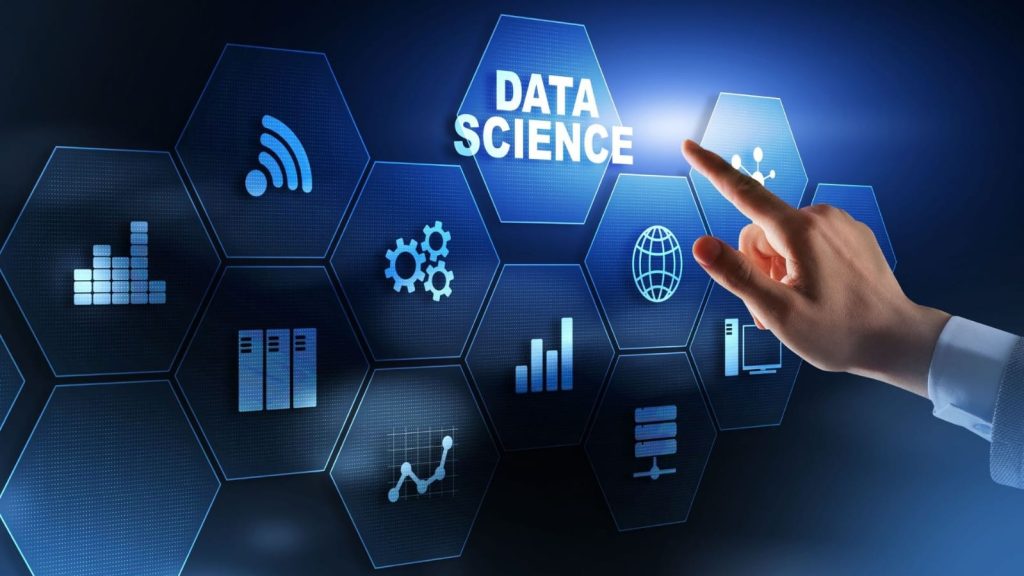
Again, when it comes to companies, they want proof that you actually have the skills you claim to have on your resume. All this requires a lot of discipline and commitment. And if you are the type of person who has or can quickly build up that resilience,that is perfect. But if not, being self-taught is even more difficult and time-consuming. What can make it even more difficult is the fact that it is very hard to find help. You can’t just ask a peer for help, nor do you have a mentor who can help you, guide you, and keep you accountable. This also means it is more difficult to get connections to people in industry that can help you get a job. There are, of course, solutions to all of this, like online communities or, for example, Discord. and you can also ask questions on Stack Overflow, but that is arguably just more stress for you to organize everything yourself. Again, I definitely don’t want to completely discourage you from taking this path, but I just want to give you some points to consider and set expectations. It absolutely can work. For example, a guy called Aleksa Gordic dropped out of his master’s program to learn ML in a self-taught way and did get a job at Microsoft and then even Google Mind. But no one sees the thousands of people who gave up being self-taught because it does not magically mean you can avoid spending months or years studying, depending on your current level, and it requires a certain personality and lifestyle. You need discipline, structure, andthe ability to hold yourself accountable. So now let’s look at the third and final option, which is arguably the middle ground between college and being self-taught. Okay, what if college is way too overkill for you because you perhaps already have a bachelor’s degree and want to simply pivot to data science? What if you need to work a job, can’t commit full-time to a program, and are not the person who can handle all the overhead of being self-taught? Well, in those cases and more, joining a bootcamp might be the right choice. A bootcamp offers you the best of both worlds. It is considerably more expensive than being self-taught, but is way cheaper than college, requires only part-time commitments for often less than a year, and is therefore very flexible. The curriculum is very specialized to a specific job, like data science, therefore taking less time than a more general college degree. And a bootcamp often even provides more knowledge on data science than colleges might. Another great advantage of a good bootcamp is that they should ideally offer weekly mentoring calls with amazing industry experts.
But yeah, all of these three options are valid paths to studying data science and getting a job. I personally don’t regret college and love it. But you really need to consider the pros and cons and see what suits you best. But no matter what you choose, you will have to spend time and money on actually learning, where you can, of course, make a lot of common beginner mistakes.